Data preparation is a vital step to perform reconciliation efficiently. In this step data is cleaned, transformed and organized for easy analysis. This includes tasks such as removing duplicates and inconsistencies, and converting the data into a format that can be easily uploaded to a software.
Data preparation is an important step in the reconciliation process as it can greatly impact the accuracy and performance of a business.
Without proper preparation, raw data may be difficult to reconcile as they contain errors, or have inconsistencies that can negatively impact the performance of a business. Additionally, data preparation can help improve the readability of the data by removing outliers, filling in missing values, and normalizing data.
Data preparation also helps to ensure that the data is ready for analysis, by preparing it into the format, and structure that’s needed by the software or tool you want to use. In short, data preparation is done to make sure the data is clean, accurate and usable for analysis and reconciliation, which leads to more accurate results.
Problems faced in data preparation
There are several problems that can arise when preparing data for accurate and efficient reconciliation
- Data quality issues: Inconsistencies, errors, or missing data in the source systems can make it difficult to accurately match and reconcile transactions.
- Data format and structure issues: Data from different sources may be in different formats or have different structures, which can make it difficult to combine and reconcile the data.
- Data duplication: Duplicate records can make it difficult to accurately match and reconcile data, leading to errors or inconsistencies.
- Data integration: Integrating data from different source systems can be a difficult and time-consuming process.
- Data security and privacy: Ensuring that sensitive or confidential data is properly secured and protected during the reconciliation process is critical.
- Scalability: Large amounts of data can cause system issues and make it difficult to process and reconcile the data in a timely manner.
How to solve data preparation problems?
Data preparation is a vital step in the process of reconciliation, as it ensures the data being used is accurate and efficient. To ensure accurate reconciliation, it is important to:
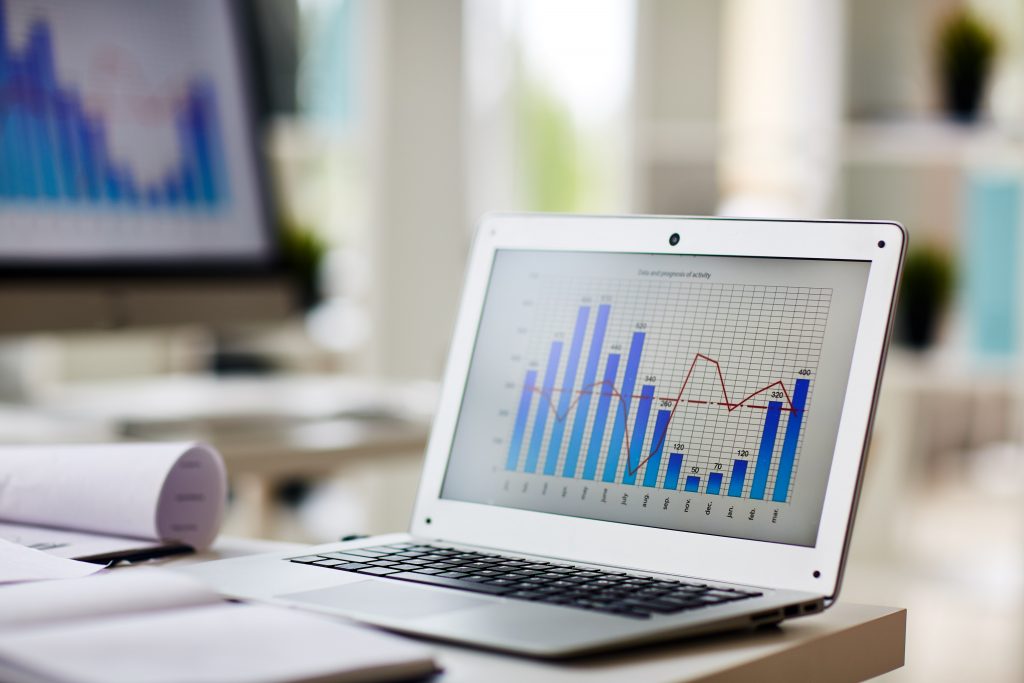
- Clean and standardize the data: Removing any duplicate or irrelevant information, and ensuring that all data is in a consistent format.
- Perform data validation: Checking the data for errors or inconsistencies, and correcting any issues that are found.
- Create a clear mapping of data fields: Identifying the correct unique identifier between different data fields, and ensuring that they are correctly linked to one another.
- Use data quality tools: Utilize tools to validate data and identify data quality issues, as well as to improve data quality through data cleaning and standardization.
- Continuously monitor and update the data: Data is never static and it’s important to regularly review and update the data to ensure its ongoing accuracy and relevance.
Ultimately, the key to solving data preparation problems for accurate reconciliation is to be thorough and to use the right tools to clean and standardize the data, and to continuously monitor and update it. Data preparation is a crucial step in the reconciliation process. By implementing these strategies, organizations can ensure that the data they are using is accurate and efficient, which will lead to more accurate and efficient business decisions.
Cointab automated reconciliation software
Cointab’s automated reconciliation software helps ease this process without having to manage or modify existing data. Data can be uploaded in any format, and the software will automatically standardize the data, clean it by removing duplicates and inconsistencies and produce results in a customized format. The data uploaded and the results are all available in one place and all the data is stored on a cloud based platform. The software offers various features which makes reconciliation seamless.